Reaching the Limit in Autonomous Racing: Optimal Control versus Reinforcement Learning was published in Science Robotics and featured on the journal’s cover. The paper presents a systematic study of control system design methodologies, focusing on reinforcement learning and optimal control in the context of autonomous drone racing. We show that neural networks trained with reinforcement learning (RL) outperform optimal control methods and trace the root cause to the flexibility afforded by RL in the formulation of the controller’s objective. In conjunction with the study, we push autonomous drones to unprecedented performance regimes, demonstrating superhuman control while reaching accelerations greater than 12g and velocities greater than 100 km/h.
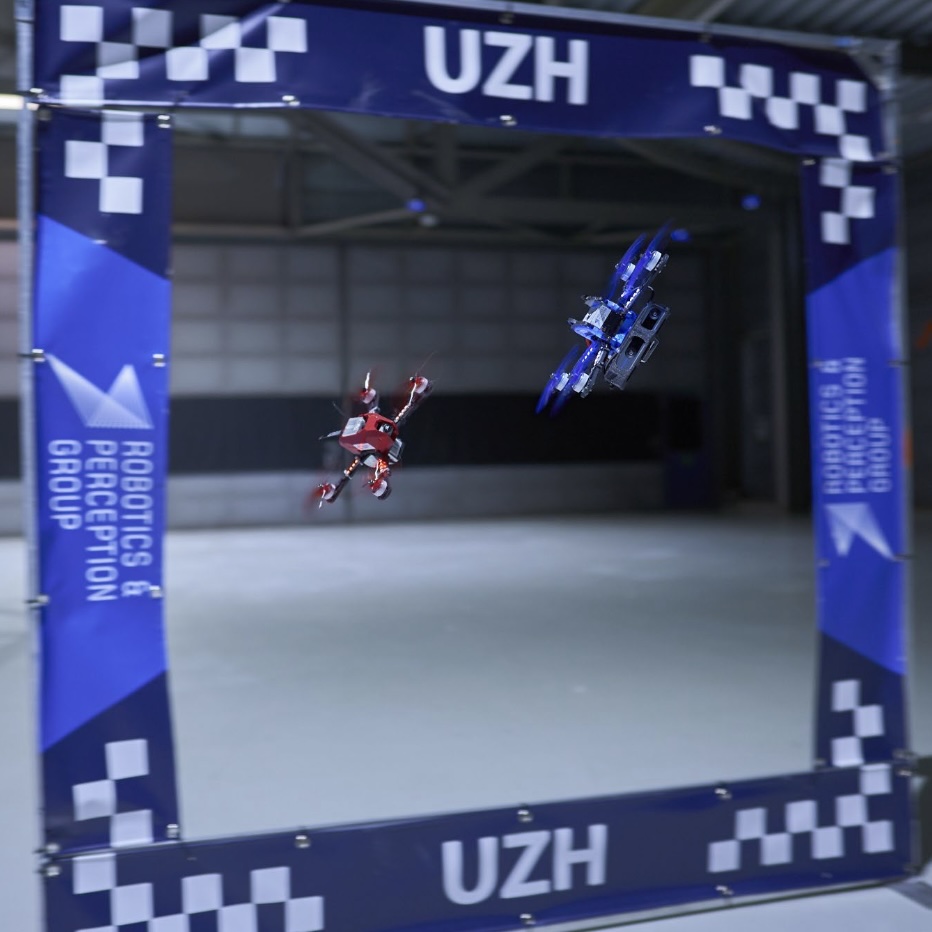
Paper published in Nature and featured on the cover
Posted on October 10, 2023
Champion-level Drone Racing using Deep Reinforcement Learning was published in Nature and featured on the journal’s cover. The paper presents an autonomous system that outraced human world champions in first-person view (FPV) drone racing, a televised sport. This is the first time that an autonomous mobile robot achieved world-champion-level performance in a real-world competitive physical sport. Here is a video created by Nature to highlight the work and here is an accompanying article that provides a good overview.