This paper shows that when applying machine learning to digital zoom, it is beneficial to operate on real, RAW sensor data. Existing learning-based super-resolution methods do not use real sensor data, instead operating on processed RGB images. We show that these approaches forfeit detail and accuracy that can be gained by operating on raw data, particularly when zooming in on distant objects. The key barrier to using real sensor data for training is that ground-truth high-resolution imagery is missing. We show how to obtain such ground-truth data via optical zoom and contribute a dataset, SR-RAW, for real-world computational zoom. We use SR-RAW to train a deep network with a novel contextual bilateral loss that is robust to mild misalignment between input and outputs images. The trained network achieves state-of-the-art performance in 4X and 8X computational zoom. We also show that synthesizing sensor data by resampling high-resolution RGB images is an oversimplified approximation of real sensor data and noise, resulting in worse image quality.
Zoom to Learn, Learn to Zoom
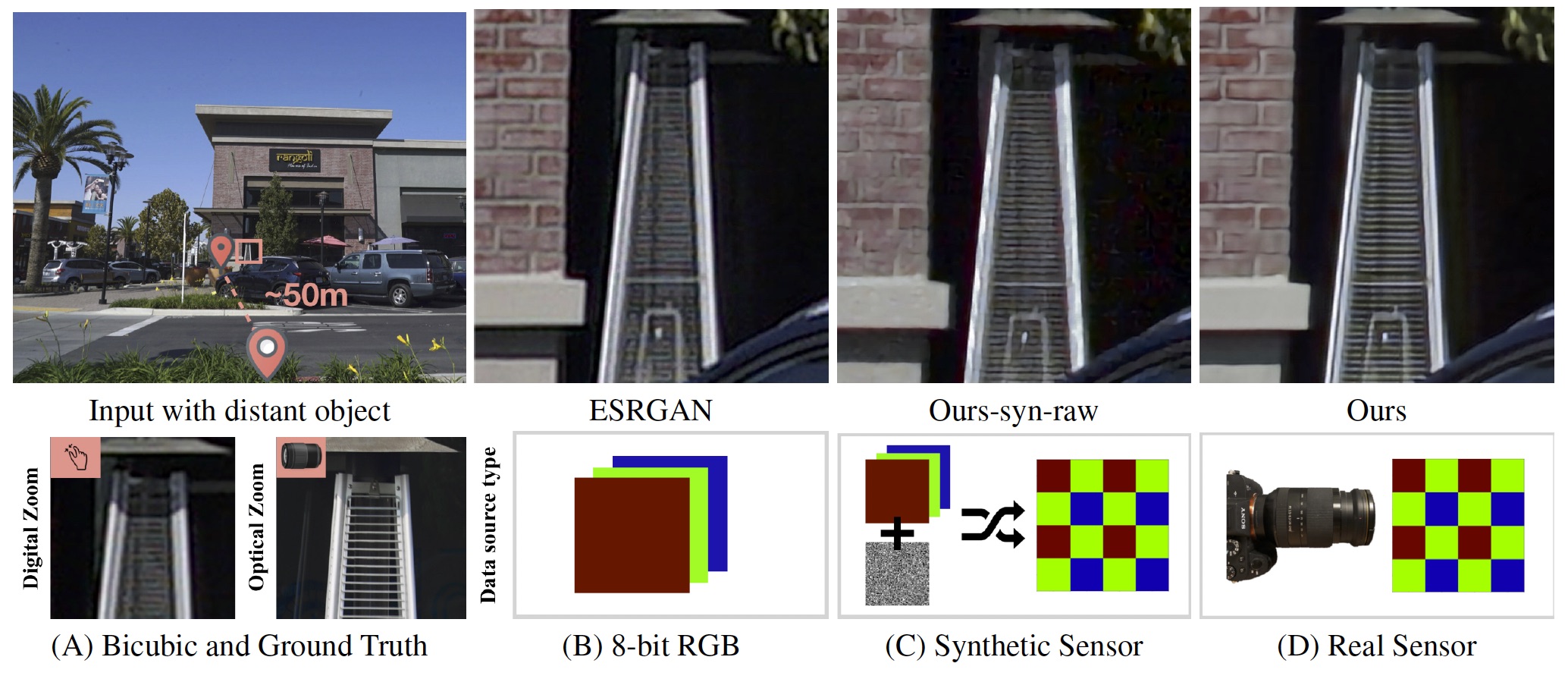